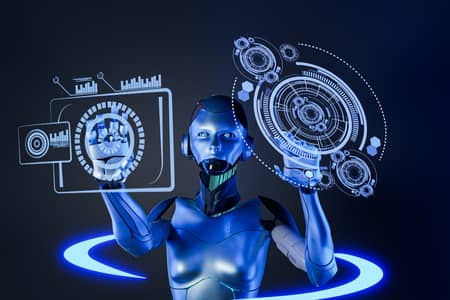
Applied Quantum computing is a rapidly developing field of computing that has the potential to revolutionize the way we solve complex problems. Unlike classical computing, which relies on binary digits or bits that can only have two values (0 or 1), quantum computing uses quantum bits or qubits, which can exist in multiple states simultaneously, allowing for faster and more efficient computations.
In this beginner’s guide, we’ll take a closer look at the key concepts of quantum computing and explore some of its potential applications.

What is Quantum Computing?
Quantum computing is a type of computing that uses quantum mechanics to perform certain calculations more efficiently than classical computers. While classical computers store and manipulate data using bits that can only have two values (0 or 1), quantum computers use qubits, which can exist in multiple states simultaneously, known as superposition.
This allows quantum computers to process many possible solutions to a problem simultaneously, which can significantly speed up certain types of calculations.
Another key feature of quantum computing is entanglement, a phenomenon in which two or more quantum particles become correlated in such a way that the state of one particle is dependent on the state of the other, regardless of the distance between them.
This property can be used for secure communication through a process called quantum key distribution.
Potential Applications of Quantum Computing
Quantum computing has the potential to revolutionize computing by providing faster and more efficient solutions to complex problems. Here are some potential applications of quantum computing:
Cryptography: Applied Quantum Computing
The emergence of quantum computing has not only raised concerns about the security of traditional cryptographic systems but has also opened up new possibilities for enhancing data protection. Applied quantum computing, the utilization of quantum principles in the field of cryptography, has the potential to revolutionize data security and enable advanced cryptographic techniques.
In this section, we will explore how cryptography is being transformed by applied quantum computing, highlighting its impact on encryption, key exchange, and secure communication.
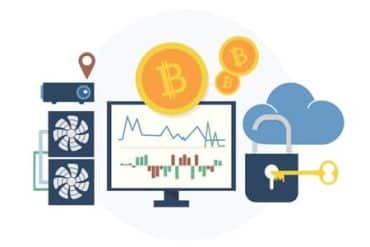
Quantum Encryption:
One of the key applications of applied quantum computing in cryptography is quantum encryption. Traditional encryption algorithms rely on the computational complexity of mathematical problems, making them susceptible to attacks from powerful quantum computers.
Quantum encryption, on the other hand, utilizes the principles of quantum mechanics to secure data transmission.
Quantum encryption leverages the phenomenon of quantum entanglement, where the quantum states of particles become intertwined. Through the use of entangled particles, known as qubits, quantum encryption ensures the security of data by exploiting the fundamental properties of quantum mechanics.
Any attempt to intercept or observe the qubits would result in a disruption of the entanglement, alerting the parties involved and rendering the communication secure.
Quantum Key Distribution (QKD)
Key distribution is a critical aspect of cryptography, as it involves securely sharing encryption keys between parties. Traditional key exchange methods are vulnerable to eavesdropping and man-in-the-middle attacks. However, QKD, a prominent application of applied quantum computing, offers a solution to these security concerns.
QKD enables the distribution of encryption keys using quantum principles. By utilizing the properties of quantum mechanics, such as the uncertainty principle, QKD ensures that any attempt to intercept the key would introduce detectable disturbances.
The receiving party can then verify the integrity of the key by measuring the quantum states of the qubits. If the key has been tampered with, the disturbance caused during interception would be readily apparent, guaranteeing secure key exchange.
Post-Quantum Cryptography
While applied quantum computing offers exciting possibilities for enhancing data security, it is essential to address the vulnerabilities of traditional cryptographic systems in the face of powerful quantum computers.
Post-quantum cryptography (PQC) focuses on developing cryptographic algorithms that are resistant to attacks from both classical and quantum computers.
PQC explores alternative mathematical problems that are computationally difficult even for quantum computers.
These new algorithms aim to replace the vulnerable cryptographic systems currently in use. Extensive research is being conducted on lattice-based cryptography, code-based cryptography, multivariate cryptography, hash-based cryptography, and other approaches that offer resistance against quantum attacks.
Quantum-resistant cryptographic algorithms are designed to withstand the computational power of quantum computers, ensuring the long-term security of sensitive data.
Transitioning to post-quantum cryptographic systems requires careful consideration and coordination among industry stakeholders to ensure a smooth migration process.
Drug Discovery: Applied Quantum Computing
The field of drug discovery is a complex and time-consuming process that holds the potential to revolutionize healthcare. With the emergence of applied quantum computing, scientists are exploring new avenues to accelerate and optimize the drug discovery process.
Quantum computing offers computational power beyond the capabilities of classical computers, enabling the exploration of large chemical spaces, molecular simulations, and the design of novel pharmaceutical compounds.
In this section, we will explore how applied quantum computing is transforming drug discovery, its applications in molecular modeling, virtual screening, and the challenges faced in this exciting field.
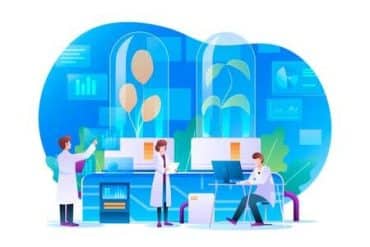
Quantum Simulations and Molecular Modeling
Quantum computing enables highly accurate simulations of molecular systems, providing insights into the behavior and properties of molecules at the quantum level. Traditional computational methods struggle with the complexity of simulating large systems due to the exponential growth in computational resources required.
However, quantum simulations allow researchers to model quantum interactions more effectively, shedding light on intricate chemical processes that are essential for drug discovery.
By harnessing quantum algorithms, applied quantum computing can accurately predict molecular properties, such as electronic structure, energy levels, and reaction mechanisms. This information guides researchers in understanding the behavior of drug molecules, optimizing their properties, and predicting their efficacy.
Quantum simulations also aid in studying the effects of environmental factors, such as solvents and temperature, on molecular interactions, providing crucial insights for drug design and formulation.
Virtual Screening and Drug Design
Virtual screening plays a crucial role in identifying potential drug candidates from vast libraries of compounds. Traditional methods rely on computationally intensive calculations to evaluate the binding affinity between a drug candidate and its target.
However, quantum computing offers the potential to significantly enhance virtual screening techniques by exploring a larger chemical space and improving accuracy in predicting molecular interactions.
Applied quantum computing can perform quantum chemistry calculations that take into account the quantum nature of chemical bonding and electron distribution. This allows for more accurate modeling of molecular interactions, enabling better predictions of drug-target binding affinities and specificity.
By efficiently exploring the vast chemical space using quantum algorithms, researchers can identify novel drug candidates with improved efficacy and reduced side effects.
Challenges and Opportunities
While applied quantum computing holds immense promise for drug discovery, several challenges need to be addressed. One significant challenge is the need for error correction and the reduction of noise in quantum computations.
Quantum systems are susceptible to errors due to decoherence and environmental interactions, which can impact the reliability and accuracy of calculations. Overcoming these challenges requires advancements in quantum hardware and error correction techniques to ensure the reproducibility of results.
Another challenge lies in the integration of quantum algorithms with existing computational workflows and infrastructure. Researchers need to bridge the gap between classical and quantum computing, developing hybrid approaches that leverage the strengths of both systems.
Collaborations between experts in quantum computing, chemistry, and pharmaceutical sciences are vital to harness the full potential of applied quantum computing in drug discovery.
Optimization Problems: Applied Quantum Computing
Optimization problems are pervasive in various real-life scenarios, spanning industries such as logistics, finance, energy, and transportation. These problems involve finding the best solution from a vast number of possible options, considering multiple constraints and objectives.
While classical computers have made significant progress in solving optimization problems, applied quantum computing offers a new paradigm that holds the potential to revolutionize optimization techniques.
In this section, we will explore real-life examples of optimization problems and how applied quantum computing can help overcome their challenges.
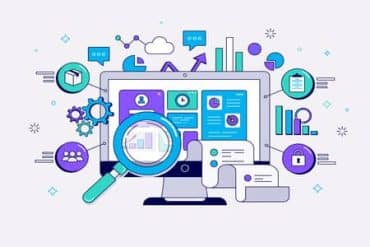
Supply Chain Optimization
Efficient supply chain management is crucial for businesses across industries. Optimization problems arise when determining the best allocation of resources, inventory levels, and transportation routes to minimize costs and maximize efficiency.
Traditional approaches to supply chain optimization often rely on heuristics and approximations due to the complexity of the problem.
Applied quantum computing can tackle supply chain optimization by leveraging its ability to handle vast amounts of data and evaluate numerous variables simultaneously.
Quantum algorithms can explore various combinations of supply chain variables, such as warehouse locations, inventory levels, and transportation routes, to identify optimal solutions that minimize costs and improve overall performance.
By utilizing the power of quantum computing, businesses can optimize their supply chains, reduce inefficiencies, and enhance customer satisfaction.
Portfolio Optimization
In finance, portfolio optimization involves selecting an optimal mix of investments that maximize returns while considering factors such as risk tolerance and market volatility.
Traditional portfolio optimization techniques rely on statistical models and mathematical algorithms that can be computationally demanding, especially when dealing with large investment portfolios.
Applied quantum computing offers the potential to improve portfolio optimization by analyzing numerous investment options simultaneously.
Quantum algorithms can efficiently explore the vast solution space, considering multiple investment combinations and risk factors. This enables investors and asset managers to make more informed decisions that balance risk and return, leading to better investment strategies and improved portfolio performance.
Energy Grid Optimization
The efficient management of energy grids is essential to ensure reliable and sustainable energy distribution. Optimization problems arise when determining the optimal configuration of energy sources, grid infrastructure, and power flow to minimize costs, reduce carbon footprint, and maintain system stability.
Traditional energy grid optimization approaches often face challenges due to the nonlinear and complex nature of power systems.
Applied quantum computing can address energy grid optimization problems by solving large-scale optimization models in real-time.
Quantum algorithms can analyze various factors, such as energy demand, renewable energy availability, transmission losses, and grid stability, to find optimal solutions that maximize energy efficiency and minimize costs.
By leveraging applied quantum computing, energy grid operators can optimize their systems, integrate renewable energy sources effectively, and contribute to a more sustainable future.
Machine Learning: Applied Quantum Computing
Machine learning, a powerful field of artificial intelligence, has found applications in various domains, revolutionizing the way we analyze data and make predictions.
When combined with the emerging field of applied quantum computing, machine learning opens up new possibilities for enhancing quantum algorithms, optimizing quantum systems, and solving complex real-world problems.
In this section, we will explore the intersection of machine learning and quantum computing, highlighting its potential and providing a real-life example of its application.
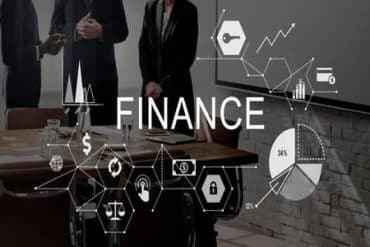
Enhancing Quantum Algorithms with Machine Learning
Quantum algorithms, such as quantum state preparation, optimization, and sampling, form the foundation of quantum computing. However, designing and optimizing these algorithms can be challenging due to the intricacies of quantum systems and the need for efficient resource allocation.
This is where machine learning techniques can play a significant role.
Machine learning can aid in the optimization and refinement of quantum algorithms by leveraging its ability to extract patterns and insights from large datasets.
By training machine learning models on quantum simulation data or experimental results, researchers can identify trends and patterns that can inform the development of more efficient and robust quantum algorithms.
Machine learning algorithms can also assist in error correction, noise reduction, and improving the fidelity of quantum computations, leading to more reliable quantum systems.
Real-Life Example: Quantum Machine Learning for Drug Discovery
One compelling real-life application of machine learning in the field of applied quantum computing is in drug discovery. Drug discovery involves analyzing vast amounts of data, such as molecular structures, biological interactions, and pharmacological properties, to identify potential drug candidates.
Traditional methods rely on extensive experimentation and simulations, which can be time-consuming and computationally demanding.
Quantum machine learning combines the strengths of both quantum computing and machine learning to accelerate drug discovery processes. Quantum machine learning algorithms can analyze molecular data and identify crucial features that impact drug efficacy, toxicity, and interactions with biological targets.
By leveraging quantum simulations and machine learning models, researchers can predict molecular properties, optimize drug candidates, and significantly reduce the time and cost involved in the drug discovery pipeline.
Quantum machine learning can also aid in the identification of novel drug-target interactions, enabling the discovery of new therapeutic targets and the repurposing of existing drugs.
By training machine learning models on quantum-generated molecular data, researchers can uncover hidden patterns and relationships, guiding the development of innovative treatments for various diseases.
Financial Modeling: Applied Quantum Computing
Financial modeling plays a crucial role in analyzing and predicting market trends, risk assessment, investment strategies, and decision-making in the world of finance.
With the advent of applied machine learning, financial modeling has seen significant advancements, enabling more accurate predictions, improved risk management, and enhanced portfolio optimization.
In this section, we will explore the intersection of machine learning and financial modeling, highlighting its potential and providing real-life examples of its application.
Improved Predictive Analytics
Machine learning algorithms excel at analyzing large volumes of historical financial data to identify patterns, correlations, and trends that may not be readily apparent to human analysts.
By training machine learning models on past market data, financial models can make more accurate predictions about future market movements, stock prices, and asset returns.
For example, machine learning algorithms can analyze historical price data, market sentiment, news articles, and other relevant information to predict stock price movements.
These models can capture complex relationships and nonlinear patterns in the data, providing traders and investors with valuable insights to make informed decisions.
Risk Assessment and Management
Risk assessment is a critical component of financial modeling, as it helps investors and financial institutions evaluate potential risks associated with investment portfolios, loans, and other financial instruments.
Machine learning algorithms can enhance risk assessment by analyzing historical data, macroeconomic factors, credit ratings, and other relevant variables to identify potential risks and forecast their probabilities.
By utilizing machine learning techniques, financial models can detect anomalies, outliers, and unusual patterns that may indicate fraudulent activities or market manipulation. This aids in fraud detection, anti-money laundering efforts, and enhancing the security and integrity of financial transactions.
Portfolio Optimization
Machine learning algorithms are invaluable in portfolio optimization, where the goal is to construct investment portfolios that maximize returns while minimizing risks. Traditional portfolio optimization techniques rely on mathematical models that make simplifying assumptions, limiting their effectiveness in capturing the complexities of real-world financial markets.
Applied machine learning can enhance portfolio optimization by considering a broader range of variables, such as volatility, correlation, liquidity, and market conditions.
By training machine learning models on historical market data, financial models can identify hidden patterns, nonlinear relationships, and interdependencies among different assets, enabling the construction of more diversified and resilient portfolios.
Real-Life Example: High-Frequency Trading
One prominent real-life application of machine learning in financial modeling is high-frequency trading (HFT). HFT relies on the use of sophisticated algorithms and machine learning models to execute trades at extremely high speeds, leveraging market inefficiencies and price discrepancies that exist for short periods.
Machine learning algorithms can analyze vast amounts of market data in real-time, detecting patterns and trends that human traders may overlook.
By incorporating machine learning into financial models, HFT firms can make rapid trading decisions, identify arbitrage opportunities, and execute trades with minimal latency, resulting in increased profitability.
Challenges and Limitations
While quantum computing has the potential to revolutionize computing, it is still in its early stages of development, and there are several challenges and limitations that need to be overcome before it can be widely used in practical applications.
One of the main challenges is the fragile nature of qubits, which are easily disturbed by external factors such as temperature and electromagnetic radiation. This requires sophisticated control and error correction techniques to maintain the coherence of the qubits.
Another challenge is scalability. While quantum computers have been built with a small number of qubits, building larger quantum computers is a complex and challenging task, and scaling up quantum computers is a major area of research.
Conclusion
Quantum computing is a rapidly developing field of computing that has the potential to revolutionize the way we solve complex problems. By using the principles of quantum mechanics, quantum computing can provide faster and more efficient solutions to problems that are difficult to solve using classical computing.
While quantum computing is still in its early stages of development, it is an exciting area of research with enormous potential for practical applications in a wide range of fields.
You can also see other articles on our website and learn more about quantum computing and you can also help us to promote education in Pakistan by buying product through our referral link of amazon.
link
Leave a Reply